Modeling Strep Throat Detection, Infection, and Spread Using an SIR Model and the Vensim Simulation Software
Editor: Susan Merkel
Published online:
Abstract
The overarching goal of this lesson is to provide students with an opportunity to gain experience making and using biological models. Biological modeling can be used to investigate complex, real-world problems so that predictions can be made about what may occur under a variety of conditions. In this exercise students generate a model for Group A Streptococcus pyogenes (strep throat) detection, infection, and spread. The lesson begins by having students investigate a common method for screening people for a strep throat infection; the rapid strep identification kit. Next, students are introduced to biological modeling by using an open source program called Vensim to model strep throat detection, infection, and spread. Students build a simple Susceptible Infected Recovered (SIR) model to simulate a strep throat outbreak at their institution. The main goal is to determine the most effective approach to control the outbreak. Students have the opportunity to assess the utility of biological modeling and recognize how a small change in the infection rate, recovery rate, or the number of initial cases can impact the total number of people at their institution who acquire the disease and how long the outbreak persists. The lesson and model can be tailored for other infectious diseases, and is amenable to several different undergraduate biology courses.
Primary image: Modeling an outbreak of strep throat. Students in this lesson make SIR (Susceptible/Infected/Recovered) models representing a strep throat outbreak with different infection and recovery rates. The picture shows the SIR model and a graph comparing the number of patients infected using data generated with 3 different models.
Citation
Kurt RA, Liew CW. 2020. Modeling strep throat detection, infection, and spread using an SIR model and the Vensim simulation software. CourseSource. https://doi.org/10.24918/cs.2020.36
Lesson Learning Goals
After this activity students should be able to:
- explore fundamental concepts and factors that influence the acquisition and spread of infectious diseases.
- conceptually describe, develop, and use biological modeling and simulation.
- generate and analyze graphs and tables.
Lesson Learning Objectives
After this activity students should be able to:
- predict the outcome of an outbreak due to changes in the factors that influence the acquisition and spread of infectious disease.
- use a Vensim biological model to investigate the acquisition and spread of infectious disease.
- generate and interpret output from a Vensim model.
Article Context
Course
Article Type
Course Level
Bloom's Cognitive Level
Vision and Change Core Competencies
Vision and Change Core Concepts
Class Type
Class Size
Audience
Lesson Length
Pedagogical Approaches
Principles of How People Learn
Assessment Type
INTRODUCTION
Because many biology curricula focus on content some students fail to grasp connections within and between systems. This disconnect provides a compelling to reason to incorporate computation and modeling skills into the undergraduate biology curriculum. The introduction of computation and modeling skills provides a framework for students to acquire a systems biology perspective. Modeling forces students to consider how components within a system affect the system as a whole as well as how one system impacts another system. It is for some of these reasons that Vision and Change (1) recommended the ability to use modeling and simulation as a core competency that every biology student should attain.
Yet, engaging biology students in computation and modeling remains a challenge. Although courses in computational biology and bioinformatics have become more common in undergraduate biology curricula, some biology students still shy away from these courses despite their importance, and many biology students seem more prone to enroll in a cell biology course rather than bioinformatics, or a molecular biology course rather than computer programming. In assessing our own majors the most common reason students reported for avoiding computational-based courses was a lack of interest, suggesting that we need to do a better job conveying the importance and exciting nature of computation-based courses. In addition to hiring faculty with expertise in computational biology to ensure students have the ability to take computation-based courses, all biology faculty can foster student interest in computation and modeling. Biology courses may incorporate a module that aims to help students appreciate the importance of computation and modeling skills in a range of disciplines. For example, immunology courses can cover the susceptible-infected-recovered (SIR) model, ecology courses can cover the predator-prey model, and molecular biology and genetics courses can cover gene regulatory networks (2-4). One advantage of this approach is the ability to show students that computational skills can be used to address a wide range of biological problems. There are also some excellent tools that allow students to create their own models such as the Vensim simulation software, and resources such as Netlogo, STELLA, iTalk, and Cell Collective that allow students to gain experience with modeling complex systems (5,6). The modeling approach also tackles the myth (and allays fears) that computation requires that students learn programming. Modeling only requires that students understand how to use computational tools, and they do not need to write programs to use them.
Here we describe a lesson developed to provide students with experience in biological modeling. We focused on the SIR model because many students find the acquisition and spread of infectious diseases a timely and interesting problem to address. We chose strep throat as the disease of interest because many of our students get tested for strep throat throughout the academic year making the topic particularly relevant to their lives. As described in the discussion, however, this lesson could easily be adjusted to investigate the spread of other infectious diseases such as influenza or coronavirus. Vensim was chosen as the modeling software because it is easy to use and it is available for free download. The lesson begins with a discussion of infectious diseases with a focus on strep throat. Students have the option to conduct a rapid strep identification test on each other; mainly to underscore the time required to diagnose a patient with strep throat. The instructor then guides students through the steps needed to make and run an SIR model. Students are required to modify their models to reflect what happens if an outbreak of strep throat occurs at their institution and no steps are taken to identify those infected; if the rapid strep identification kit is used to identify those infected; or if a traditional microbiological method is used to identify those infected. Students are required to generate three different models, and analyze and compare the data generated with each model. This lesson does not require much background knowledge making it amenable to several different undergraduate biology courses including immunology, infectious diseases, microbiology, and general biology.
Intended Audience
The lesson was designed as a lab for a 200 level immunology course for undergraduate biology majors (sophomores through seniors) at a liberal arts college. Although we typically teach this lesson later in the semester following a chapter on infectious diseases, the lesson was primarily designed to provide students some experience with modeling and simulation, not to teach immunology. The lesson could also be used for courses in microbiology, infectious diseases, and general biology.
Required Learning Time
The lesson was designed to run during a 3 hour lab session, but could be modified for one or two one-hour lecture sessions.
Prerequisite Student Knowledge
A basic understanding of the acquisition and spread of an infectious disease is helpful, but not required. No experience with biological modeling is required. Students create models without having to learn a programming language or write a program. However, students should be able to use computer programs and have experience with Excel. Depending on the course, and what the instructor wants to focus on for this exercise, the instructor may want to provide some understanding of microbes and microbial infections especially the disease-causing organism, Group A Streptococcus.
Prerequisite Teacher Knowledge
A basic understanding of acquisition and spread of infectious diseases is helpful. We find the Centers for Disease Control and Prevention is an excellent source for up-to-date information about Group A Streptococcal disease (https://www.cdc.gov/groupastrep/index.html). No experience with biological modeling is required. The lesson includes step-by-step instructions for creating and using an SIR model with the Vensim software, although there are excellent resources about modeling infectious diseases for instructors who may want to learn more (7-9).
SCIENTIFIC TEACHING THEMES
Active Learning
The lesson was designed as an active learning experience. Students conduct a strep throat test and, using a computational model that they build, are able to address questions related to the acquisition and spread of infectious disease. Once students begin running their models and generating data, the class is able to discuss the implications of their findings.
Assessment
Once students have generated a functional model they are able to export their data into table or graph formats and analyze their data in order to answers questions related to the acquisition and spread of disease. Questions could focus on whether students were able to acquire and interpret their data and should also include more open ended questions such as:
- At the peak of the outbreak how many students were infected with strep throat?
- Why may testing for strep throat prolong an outbreak?
Inclusive Teaching
The lesson is amenable to discussions about equity in health care. For instance, the instructor can ask students to model what happens if those infected are not isolated or treated because they lack health care and/or work benefits such as paid sick leave.
LESSON PLAN
Pre-Lab
We make Part I (i.e., have students conduct the rapid strep identification kit) optional for the students. The main reason to perform Part I is to underscore the time needed to perform the test and obtain a diagnosis. Of note, this can also be accomplished with a discussion of the test. While most of our students perform this test, some students are not comfortable having their throat swabbed. Instructors with little or no experience in conducting throat swabs, dealing with human samples, and without a strong background in laboratory safety should consult appropriate resources such as ASM Guidelines for Biosafety in Teaching Laboratories, before considering this optional aspect of the lesson. If you are going to run Part I of the lesson then make sure the Sure-Vue strep A test (Fisher Scientific cat# 23-200-276) has been ordered and you are familiar with the test. It is also a good idea to download Vensim and familiarize yourself with the steps needed to construct and run the SIR model.
Strep Throat Testing and Modeling an Epidemic: Part I. Testing for a Strep Throat Infection
Rapid Identification Kit
If you have ever been tested for strep throat it is likely the health care provider used a rapid strep identification kit similar to the Sure-Vue strep A test. This immunoassay makes use of an antibody to detect antigen(s) on Group A Streptococcus pyogenes from a throat swab. Antibodies, which are proteins produced by a type of white blood cell called a plasma cell during an immune response, are extremely specific for substances (proteins, carbohydrates, etc., generally referred to as antigens) present on pathogens; much like an enzyme is specific for a substrate. In the case of an immune response the antibody would be like the enzyme and the substrate would be like the antigen. Antibody-based immunoassays, such as the Sure-Vue strep A test, are extremely specific; the antibody used in this test will only recognize antigen on Group A Streptococcus pyogenes, the causative agent of strep throat. The immunoassay results in a color change when the antibody binds to the antigen found in Group A strep and as a result, the test is simple to read and requires no elaborate equipment and supplies.
Some advantages of this test include the fact that it is fast to perform (a few minutes), relatively inexpensive, stable at room temperature, and is 85% sensitive and 100% specific. Some disadvantages of the test include the fact that the test may give a positive result even if the bacteria are dead, the test can be negative if the throat swab is not taken properly, and the test does not differentiate carriers from those with acute infection. For this part of the lesson students can swab each other's throats and perform the rapid strep identification test. Make sure students run their sample(s) as well as the positive and negative controls that come with the kit. Detailed step-by-step instructions for the Sure-Vue strep A test are provided with the kit.
Microbiological culture
The identification of many bacterial human pathogens can begin with growing a culture of the specimen on selective or enrichment media. The general idea is that if you know what symptoms the person has then you can make an educated guess about what is causing the disease and therefore try to grow that particular organism. In the case of a sore throat, group A strep may be suspected. Although microbiological testing is not common for patients suspected of having strep throat, you should understand how this test works as well as the advantages and disadvantages of using microbiological testing for a strep throat infection.
It is known that group A strep will grow on blood agar plates and give an area of β-hemolysis (a clear area surrounding the bacteria) because the bacteria lyse the blood in the media. Many organisms do not grow on blood agar plates such as staphylococci, Neisseria, and other gram (-) bacteria. Similar to the rapid strep identification kit this test begins with a throat swab. A throat swab is used to streak a blood agar plate. After the plate has incubated for 24 hours at 37°C the plate is observed for colony size and area of hemolysis. Group A strep will appear as small white colonies with a large area of β-hemolysis. However, the test does not end here. The presence of small white colonies and β-hemolysis are indicative of Group A strep, but additional studies would be needed to verify whether the organism is indeed group A strep. These additional procedures could take another 24 hours. The advantages of this test include the fact that the test is relatively cheap, and the test is 95% sensitive and 100% specific. Disadvantages include the fact that the test requires a minimum of 24 hours to grow the bacteria, and the test should be coupled with additional tests to verify the species of bacteria requiring even more time.
Strep Throat Testing and Modeling an Epidemic: Part II. Modeling Strep Throat Detection, Infection, and Spread
Biological modeling can be used to investigate complex, real-world problems so that predictions can be made about what may occur under a variety of conditions. In this exercise students will generate a model for strep throat detection, infection, and spread using an open source program called Vensim. To download a copy of Vensim go to vensim.com, select Download, and then choose the Free Download for educational purposes.
Information about the S (susceptible) → I (infected) → R (recovered) model
Students will go through the step-by-step process of making an SIR model. Although there are many different ways to model biological processes, one of the first things to learn (after learning the software) is that it is always best to start with a simple model. Students need to determine the major factors that need to be taken into account for the initial model. Students also learn how to differentiate between primary, secondary, and tertiary factors. Primary factors include the bare minimum needed to make the model. For instance, the factors used in this lesson, three groups of people (susceptible, infected, recovered), the infection rate, and the recovery rate, are primary factors. A secondary factor would include a factor added to make the model more sophisticated once the model is functional with the primary factors. For instance, perhaps some of the susceptible people are genetically resistant to the disease or have been protected by vaccination. Or, perhaps not all of those infected with the disease recover but die instead, or become asymptomatic carriers of the disease. A tertiary factor would include a factor added to make the model even more sophisticated once the model is functional with a secondary factor. For instance, perhaps those who recovered do not have immunity and therefore become susceptible again, or perhaps some of the susceptible people engage in behaviors that put them at greater risk of acquiring the infection.
The initial model should only consider primary factors. Once that is complete and tested, students can expand the model to include secondary factors. In other words, once students generate a simple model that works they can then use the model as a scaffold to build more sophisticated models capable of addressing more complex problems, although this is beyond the scope of the current lesson. Here, students build a simple SIR model to address the question: Is the rapid strep identification kit or traditional microbiological procedures more effective in controlling an outbreak of strep throat at their institution? This question is important to address because the strep identification kit is not as sensitive as the microbiological method, but the culturing method takes longer.
Make sure the students have Vensim on their computers and are ready to begin. (Supporting File S1. Modeling Strep Throat – Step by Step Instructions for Generating an SIR Model).
During the lesson, students will build the model, then run it. Students will investigate variations of the basic model, based on the type of diagnostic test, then graph each variation, and compare the data generated by the different models.
For any type of model a number of assumptions are needed. Below are some assumptions that have been made for the basic model described in this lesson.
- All Susceptible people become infected.
- Infected people recover, and infected people can infect susceptible people.
- Nobody dies from the infection.
- The rapid strep test has a sensitivity of 85% in identifying group A strep.
- The microbiological method has a sensitivity of 95% in identifying group A strep.
Some additional assumptions we are making for the model:
- I (infected) has an initial value of 10; so 10 students are initially infected.
- S (susceptible)has an initial value of 2900; the entire student body is susceptible to infection.
- R (recovered) has an initial value of 0; nobody has yet recovered from the infection
- The Infection rate is 0.001, with a minimum value of 0.001, maximum value of 0.01, and can be increased or decreased in increments of 0.0001.
- The recovery rate is 0.2, with a minimum value of 0, maximum value of 1, and can be increased or decreased in increments of 0.01
- Infection = infection rate * S * I
- Recovery = recovery rate * I
- For the rapid strep kit Infection = infection rate * S * (I * 0.15)
- For microbiological testing the Infection = infection rate * Susceptible * (Infected * 0.05)
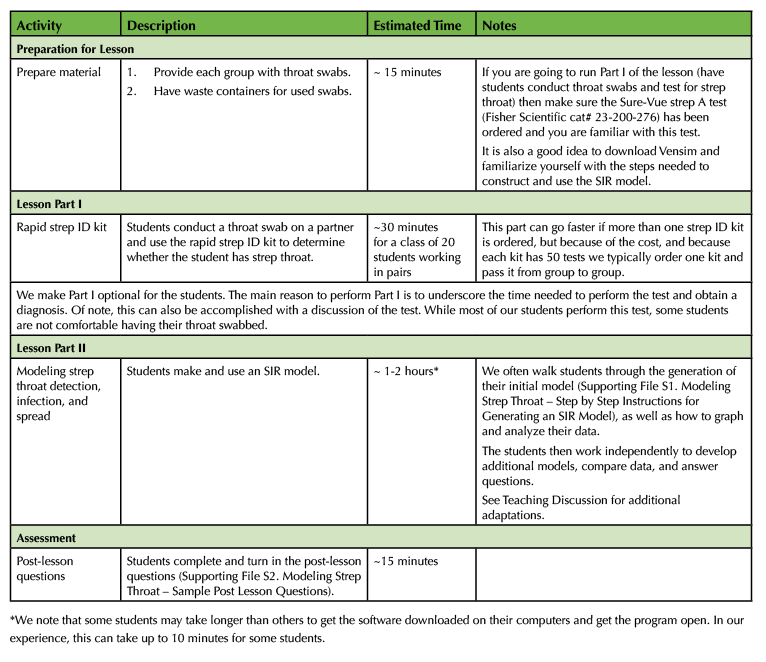
Table 1. Lesson plan timeline.
TEACHING DISCUSSION
Learning Goals and Objectives
Following this lesson students should know the information that is required to model an epidemic or pandemic, and they should understand how to make and use a biological model. The instructor could continue this lesson by having students model acquisition and spread of other infectious diseases. Many undergraduate biology courses provide experience in data analysis and this lesson is no exception. We like to have students graph the data so that they can interpret what is happening with respect to increasing or decreasing the number of individuals that are susceptible, infected, or recovered from the disease at any given time. If the instructor asks for specific data, for instance how many people were infected after 2 days of the outbreak, then students can consult data in the tables to obtain the exact number of people infected at that time point.
Floating Point Error Message
One common error message we have encountered is a floating point error message. We've found this typically happens if the projected numbers reach levels the model cannot handle. One way to avoid this error is to keep the population you are modeling relatively small. For instance, when we start with a population of 3,000 rather than 2,900 we receive a floating point error at time step 18. If students wish to model large populations we typically ask the students to think about scaling. For example, students can keep the initial population at 2,900 and if they want to know how many would be infected if they were modeling a population of 290,000 then they can multiply their data by 100.
Taking into Account a Time Delay
Sometimes an infectious disease is present for a period of time before it is identified, or before it can be confirmed by specific tests. A time delay can be used to account for this. For example, we typically use a time delay for our microbiological testing model since a diagnosis would require 24-48 hours. In this scenario we are making an assumption that during that 48 hour time period all infected people continue to spread the disease. As a simple approach to take into account this time delay we ask students to determine the number people infected at 48 hours if no screening method is used (the basic model) and then we use that number as the number of people initially infected in the new model. For example, if one follows Supporting File S1 for Step by Step Instructions for Generating an SIR Model then there are typically about 135 people infected at 48 hours. So instead of having an initial population of 10 people infected, we change the initial number to 135 in the microbiological testing model to represent the 48 hour time delay before initial diagnosis is determined. An alternative, and equally valid assumption could be that the people suspected of being infected are quarantined to prevent further spread. With this assumption made a time delay is not needed.
Possible adaptations to lesson
As mentioned earlier, the lesson can be used for several different courses and depending on the student population more or less information can be supplied at the start of the lesson. For instance, for an immunology or infectious disease course the instructor may focus on the modeling aspect of the lesson if acquisition and spread of disease was covered previously in the course. Alternatively, this lesson could be used as a problem-based learning module to cover biological modeling as well as the acquisition and spread of infectious diseases. For an introductory biology course the focus could be an introduction to computational modeling. One can also modify the model for different locations for instance taking into account spread of a disease in a nursing home or college dormitory. The instructor could also adapt the model to another infectious disease that may be in the news at the time the course is being taught. See Supporting File S3. Modeling Strep Throat – An Adaptation to Incorporate Death from the Disease, for a sample on how the model could be used for Covid-19. Time permitting, the instructor can ask students to hypothesize how altering the number of infected individuals, the infection rate, recovery rate, etc. would impact the number of people infected over time. There are plenty of opportunities to ask and address interesting questions about the acquisition and spread of an infectious disease.
SUPPORTING MATERIALS
- S1. Modeling Strep Throat – Step by Step Instructions for Generating an SIR Model
- S2. Modeling Strep Throat – Sample Post Lesson Questions
- S3. Modeling Strep Throat – An Adaptation to Incorporate Death from the Disease
References
- Brewer CA, Smith D. 2011. Vision and Change in undergraduate Biology Education a Call to Action. American Association for the Advancement of Science.
- Chowell G, Sattenspiel L, Bansal S, Viboud C. 2016. Mathematical models to characterize early epidemic growth: a review. Physics of Life Reviews, 18:66-97. doi: 10.1016/j.plrev.2016.07.005.
- Gamez M, Lopez L, Varga Z, Garay J. 2017. Ecological monitoring in a discrete-time predator-prey model. Journal Theoretical Biology, 429:52-60. doi.org/10.1016/j.jtbi.2017.06.025.
- Hecker M, Lambeck S, Toepfer S, von Someren E, Guthke R. 2009. Gene regulatory network interference: Data integration in dynamic models. Biosystems, 96:86-103. doi: 10.1016/j.biosystems.2008.12.004.
- Wang Y, Wang R, Zhang S, Song S, Jiang C., Han G, et al. 2019. iTALK: an R Package to Characterize and Illustrate Intercellular Communication. BioRxiv. doi.org/10.1101/507871.
- Helikar T, Kowal B, McClenathan S, Bruckner M, Rowley T, Madrahimov A, et al. 2012. The cell collective: Toward and open and collaborative approach to systems biology. BMC Systems Biology. 6:96. doi: 10.1186/1752-0509-6-96.
- Kramer A, Krickeberg K, Kretschmar M. 2010. Modern infectious disease epidemiology: concepts, methods, mathematical models, and public health. New York: Springer Science and Business Media.
- Jit M, Brisson M. 2011. Modelling the Epidemiology of Infectious Diseases for Decision Analysis: A Primer. Pharmacoeconomics. 29:371-86. doi: 10.2165/11539960-000000000-00000.
- Coburn BJ, Wagner BG, Blower S. 2009. Modeling Influenza Epidemics and Pandemics: Insights Into the Future of Swine Flu (H1N1). BMC Med. 7:30. doi: 10.1186/1741-7015-7-30.
Article Files
Login to access supporting documents
Modeling Strep Throat Detection, Infection, and Spread Using an SIR Model and the Vensim Simulation Software(PDF | 145 KB)
S1. Modeling Strep Throat - Step by Step Instructions for Generating an SIR Model.docx(DOCX | 2 MB)
S2. Modeling Strep Throat - Sample Post-Lesson Questions.docx(DOCX | 16 KB)
S3. Modeling Strep Throat - An Adaptation to Incorporate Death from the Disease.docx(DOCX | 17 KB)
- License terms
Comments
Comments
There are no comments on this resource.