Learning Quantitative Genetics: Investigation of Genetic Control for Cold Stress Response in Plants
Editor: Megan Barker
Published online:
Abstract
Course-embedded undergraduate research experiences (CUREs) for students have been shown to increase students’ understanding of the process of science, affirm their scientific identity, and improve retention in STEM fields. Despite many CUREs recently developed for introductory biology, genetics, biochemistry, and molecular biology courses, projects related to quantitative genetics and polygenic inheritance are rare. Students frequently struggle with the uncertainty and complexity of quantitative genetic studies in a traditional genetics course. This lesson describes a series of laboratory exercises that provide an authentic research experience focused on quantitative trait loci (QTL) analysis of the traits related to cold stress response in plants. Maize varieties show a large variation in the degree of their response to stress, suggesting that this trait is highly heritable, even though most of the genes contributing to this trait remain elusive. The results of the QTL analysis vary depending on the plant material used in the study and the specific traits measured in the study, reflecting the polygenic nature of the trait. This laboratory project allows students to make decisions about the details of the experimental design, collaborate with their peers, conduct the experiments, and analyze the results using standard protocols for the QTL analysis. The accompanying worksheets and supplemental instruction demonstrate the complex architecture of quantitative traits and their dependency on the number of plants involved in the analysis and the details of the experimental design. The laboratory series invites students to discuss the nature of the scientific investigation.
Primary image: Different Maize Varieties Show Variation in Cold Stress Tolerance. When exposed to 8 hours of 600C, B73 maize seedlings exhibit severe stress response, including dying leave edges and tips and leaf curling. Mo17 maize seedlings are resistant to cold stress under these conditions and show no stress response phenotype. The image was taken by the authors.
Citation
Makarevitch I, Goering R. 2022. Learning quantitative genetics: Investigation of genetic control for cold stress response in plants. CourseSource. https://doi.org/10.24918/cs.2022.4Society Learning Goals
Genetics
- Transmission - Patterns of Inheritance
- How can one deduce information about genes, alleles, and gene functions from analysis of genetic crosses and patterns of inheritance?
- How does the phenomenon of linkage affect the assortment of alleles during meiosis?
- Methods & Tools in Genetics
- What experimental methods are commonly used to analyze gene structure and gene expression?
Lesson Learning Goals
Students will:- observe the genetic variation between maize varieties in response to cold stress and will explore the nature and genetic control of quantitative traits.
- design and conduct simple quantitative trait locus (QTL) experiments and interpret their results using available genetic and physical genome maps.
- understand the complexity of polygenic traits and the role of gene linkage and genetic markers in the process of identifying genetic factors controlling these traits.
Lesson Learning Objectives
Students successfully completing this lesson will be able to:- explain genetic mechanisms controlling complex genetic traits.
- describe the structure of mapping populations, including recombinant inbred lines (RILs), and explain their use in gene mapping.
- perform a basic QTL analysis and explain its results.
- discuss requirement, advantages, and limitations of QTL analysis.
- analyze physical and genetic map content of maize genome regions using maize genetic database.
- Students should be able to implement observational strategies to formulate a question.
- Students should be able to design an experiment using appropriate controls and appropriate sample sizes.
- Students should be able to gather and evaluate experimental evidence, including qualitative and quantitative data.
- Students should be able to apply statistical methods when analyzing their data, and use patterns to construct a model.
- Students should be able to generate and interpret graphs displaying experimental results.
Article Context
Course
Article Type
Course Level
Bloom's Cognitive Level
Vision and Change Core Competencies
Vision and Change Core Concepts
Class Type
Class Size
Audience
Lesson Length
Pedagogical Approaches
Principles of How People Learn
Assessment Type
Introduction
National initiatives in biology education recognize the need for all biology students to participate in research (1-3). In addition to deeper understanding of biological concepts, students participating in research demonstrate increased persistence in science, increased science self-efficacy, ownership of their work, lasting science learning, and increased understanding of research processes (4-11). Authentic research experiences, from smaller investigations to full semester course-embedded undergraduate research experiences (CUREs), involve students in science research within the context of a course, thus allowing participation by a greater number of students compared to a more traditional one-on-one mentorship (12-17).
Quantitative genetics is a complex topic that receives little attention in the introductory and intermediate level genetics courses, even though many important traits, including human diseases (for example, diabetes and hypertension) and crop performance (for example, yield and nutrient composition), are controlled by multiple genetic factors in addition to environmental effects (18, 19). Measuring quantitative traits, analyzing quantitative data, and describing the influence of genetics and environment on phenotypes also exercises students’ use of quantitative reasoning, a key competency in the AAAS’ Vision & Change document (20).
Plants are frequently neglected when teaching biological sciences (21,22). The consequences for this observed lack of inclusion for plants—termed “plant awareness disparity” (23, 24)—vary, including decreased understanding of plant biology and less funding for plant-based research (25). While plant systems have been used successfully in the classroom to illustrate a variety of biological concepts in various disciplines and to engage students in research opportunities (26-30), plant-based learning experiences lag behind other popular model systems.
The series of laboratory exercises presented here allow students to engage in an original investigation of a genetic architecture of stress response in maize seedlings. It leverages publicly available maize genome sequences and genetic maps to involve students in the scientific process. Environmental stress response in plants is a highly heritable complex genetic trait affected by many genes (31,32). However, only a few genes controlling stress response have been cloned and characterized (33-35). Maize seedlings from different varieties (inbreds) display different responses to environmental stress, for example, cold exposure (Figure 1). The QTL analysis of cold stress response showed ample variation in stress response among maize lines and yielded multiple candidate loci that differed depending on the specific conditions of the stress and the trait measured (36-40). Maize genetic resources include a publicly-available population of recombinant inbred lines (RILs) with a well-characterized genetic structure for Quantitative Trait Locus analysis (QTL) (41). Thus, environmental stress response, with its variability and potential for discovery, and maize, with its publicly available genetic maps and recombinant populations, provide a good opportunity for original student research projects.

Intended Audience
This series of laboratory exercises was designed for and implemented in an introductory Genetics course in a liberal arts college (sophomore and junior students majoring in biology). This laboratory series was also used with junior and senior students majoring in biology in an upper-level genomics and bioinformatics course. Some knowledge of basic genetics is required, and a semester of introductory biology focusing on genetics is highly recommended.
Required Learning Time
The laboratory series on quantitative genetics presented here was implemented during three three-hour laboratory sessions (a total of nine student contract hours). Additionally, the plants need to be planted and subjected to the cold stress prior to the first lab session (see Supporting File S1. QTLs of Maize Cold Response – Detailed Timeline for details). Additional time was required for pre- and post-instruction assessment and student surveys. In addition, students needed extra time outside of class to complete reports of their work.
Prerequisite Student Knowledge
Students need to understand the general principles of genetics, such as independent assortment, segregation, and gene linkage, as they guide inheritance of simple genetic traits. A short introduction to quantitative genetics prior to the laboratory activities is useful for students, although an instructor could provide required background information during a Laboratory session as well. We asked students to read a relevant chapter of their textbook for an introduction to quantitative genetics and complex traits, as well as a short introduction to QTL analysis (42).
The lab description contains detailed information on how to run QTL analysis in R and the prior experience in R is not required.
Prerequisite Teacher Knowledge
The instructor should understand the general principles of the polygenic trait inheritance and QTL analysis, including the meaning and significance of the LOD scores. The instructor should practice the analysis pipeline to be able to help students as they navigate the analysis. Specifically, the faculty member should be aware of how to lead the students through the QTL analysis in R. Most common mistakes students do are related to syntaxis, such as missed or misplaced semicolons, and commas, and could be spotted quickly with some practice. We recommend the instructor considers exploring an R/QTL guide (43). Explicit knowledge of plant biology is not required, understanding that corn could be self-pollinated or cross-pollinated is useful in case students are confused.
Scientific Teaching Themes
Active Learning
During this laboratory series, students work together in small groups and engage in discussion and answer questions about principles, advantages, and limitations of quantitative trait locus mapping. They conduct phenotypic observations, make decisions about the details of experimental analysis, decide which traits they will score, and estimate the effects of cold stress on maize seedlings using quantitative and qualitative approaches they develop. Students, individually or in pairs, run quantitative trait locus mapping analysis using R software, visualize their results, interpret data, and make conclusions.
Assessment
Students’ answers to the questions in four graded worksheets (see Supporting File S2. QTLs of Maize Cold Response – Student Handout for the questions and Supporting File S3. QTLs of Maize Cold Response – Sample Answers to the Questions of the Worksheets for sample answers) were used to assess their understanding of the genetics of quantitative traits and applications and limitations of QTL analysis approaches. Students engaged in this laboratory exercise also completed pretest and graded posttest (Supporting File S4. QTLs of Maize Cold Response – Multiple Choice Assessment Quiz with Correct Answers). To assess the results of the project and validate this lab series, students presented their results in graded lab reports, where they evaluated whether the results of the QTL mapping analysis supported their hypothesis regarding the prediction of the number of major loci controlling cold tolerance trait in maize (Supporting File S5. QTLs of Maize Cold Response –Rubric Used for the Assessment of Student Skills in Data Analysis and Interpretation in the Lab Reports). For student’s subjective assessment of the effects of the laboratory series on development of various science-related skills, students completed the CURE survey (44). The CURE Survey is designed to measure student experiences in "research-like" or other science courses and is based on students’ report of learning gains and attitudes toward science through a series of Likert-type items. More information related to the CURE survey is available on CURE Survey website at Grinnell College.
Inclusive Teaching
This laboratory series provides authentic research opportunities embedded in the curriculum. While many students find summer research opportunities more demanding, highly competitive, and less accessible due to students’ obligations outside of school, course-embedded research opportunities allow all students to engage in science (16). Students with different attitudes toward science, different preferences for lab work and computational data analysis, and different levels of prior knowledge work together in small teams. This laboratory series involves investigating plant genetics, a topic frequently omitted from genetics courses, thus attempting to address “plant awareness disparity,” lack of plant-related topics in many college biology courses. Students discuss, observe, and investigate genetic diversity of maize lines and make connections to genetic diversity in human populations.
Lesson Plan
The general flow of the experiments and analysis conducted in this Lab is shown on Figure 2 (see Supporting File S1. QTLs of Maize Cold Response – Detailed Timeline for the detailed timeline for this laboratory series). The student handout including all worksheets and instructions is available as Supporting File S2. QTLs of Maize Cold Response – Student Handout.
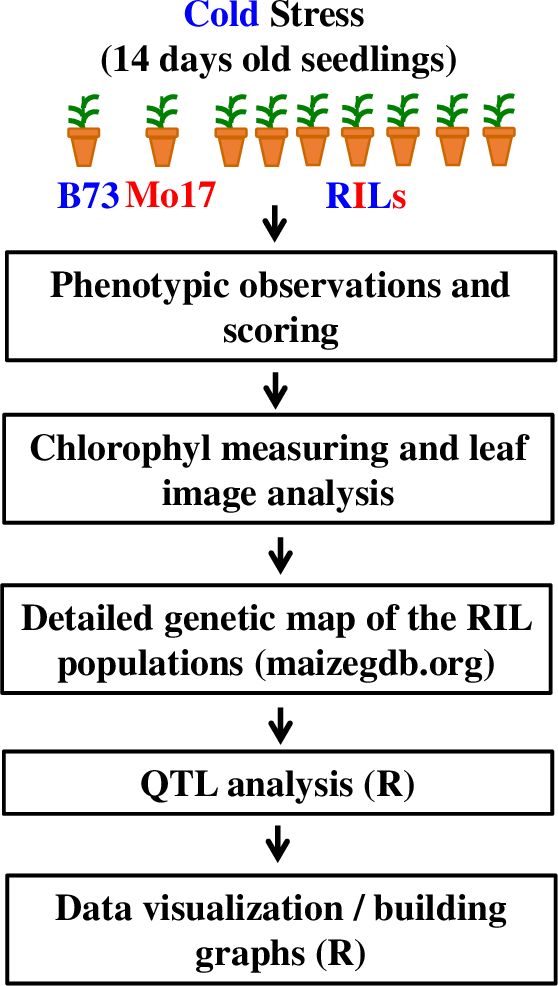
Instructor Preparation
Plant Growth and Stress Conditions
Seeds for B73 and Mo17 maize inbreds as well as seeds for B73xMo17 recombinant inbred lines are available through the Maize Genetics Stock Center. While the Stock Center only typically provides a few seeds for each of the lines, they do support undergraduate instructors and researchers and will likely provide enough seeds for this laboratory series. In addition, many maize researchers in large universities work with B73, Mo17, and RILs and should be able to provide a small number of seeds (under 10 for each line) that are necessary to conduct this lab series. We obtained all of our seeds from Dr. Nathan Springer at the University of Minnesota.
Planting of the B73 and Mo17 inbreds, as well as recombinant inbred lines (RILs) needs to be timed, so, the cold stress could be administered 10–13 days after planting. To facilitate planting, students were asked to plant seeds during the laboratory sessions two weeks prior to the corresponding lab activity (see Supporting File S1. QTLs of Maize Cold Response – Detailed Timeline for details). Adding a regular fertilizer (for example, Miracle Gro©) to the soil is beneficial, especially if soil lacks magnesium, phosphates, and other nutrients, because maize seedlings tend to show various amounts of purple color in the stems and leaves in response to lack of nutrients, and the color may influence the analysis of chlorophyll content in the leaves. Maize seedlings could be grown in growth chambers or green houses, or even under the light in regular rooms, at 20–24ºC and 16-hour light days. The cold stress implemented in this laboratory series was conducted by the instructor, one–two days before the lab, at 4ºC in the growth chamber during the night (12 hours). Alternatively, cold rooms, or even, the refrigerators, could be used for administering the stress conditions, with the stress temperature ranging between 4 and 12ºC. Stress phenotype is more pronounced, when the plants are left relatively dry before and during the stress and are allowed to recover for 1–3 days under normal conditions. To account for possible germination failure and provide sufficient replication for measurement of chlorophyll content, three–five seeds from each of the lines were planted. Some of the plants from B73 and Mo17 inbreds were not stressed to provide control measurements and observations.
Genetic Structure of the RILs and File Management
The maize intermated B73×Mo17 (IBM) recombinant inbred line population (IBM Syn4 RIL) was generated from B73 and Mo17 parental lines by four generations of intermating and eight generations of inbreeding (41) (Figure 3), resulting in about 3.5 fold higher recombination compared to F2 - derived recombinant inbred lines.

To facilitate genetic mapping by the students and to prevent confusion associated with file formatting, we created a csv file for ~2000 markers and 95 RILs used in this laboratory exercise (Supporting File S6. QTLs of Maize Cold Response – Genetic Architecture of the Lines Used in This Study). We used Google Sheets to allow students simultaneously record phenotypic observations and measurements for the RIL lines during week 2 of the Lab series (see Supporting File S7. QTLs of Maize Cold Response – Sample Phenotypic Data Collected by Students). After the phenotypic information was recorded by students in multiple lab sections (~80 students in 4 lab sections in our implementation), the instructor can calculate average values for all phenotypic observations for each line, combine genetic and phenotypic information for the RILs, and prepare the file for subsequent QTL analysis (see Supporting File S8. QTLs of Maize Cold Response – Sample Formatted Data File Ready with Visual Scoring Data for QTL Analysis).
Lab Sessions
Phenotypic Observations and Discussion of Quantitative Genetic Traits and QTL Analysis Approaches – Week 1
Prior to the session, the students read short sections on quantitative genetic traits in their textbook and receive a short introduction to the complexity of genetic control of polygenic traits. The student handout (see Supporting File S2. QTLs of Maize Cold Response – Student Handout) contains some background information that could be expanded upon the desire and comfort level of the instructor. During the first week of this lab series students are first presented with a quantitative genetic trait, plant response to cold stress. They are asked to observe and describe the response to cold stress in B73 and Mo17 seedlings and discuss the potential mechanisms of genetic control of this trait, as well as potential approaches to measuring and documenting the differences in response to cold stress between two maize inbreds. At the next step, students are asked to think about how genetic mapping works, what populations of plants are required for genetic mapping, and how the results of the crosses would depend on the genetic architecture of the mapped trait. Students realize that F2 populations are necessary for genetic mapping and draw the chromosome structure of parental, F1, and F2 generations. They also predict the segregation ratios and chromosome structure of cold tolerant and cold resistant plants in F2 populations under various assumptions about the genetic architecture of the cold resistance, from a simple one gene completely dominant trait to a more complex multi-gene trait. The main outcome of Week 1 session is to prepare students for the phenotypic and genetic analysis in weeks 2 and 3.
Phenotypic Observations and Trait Scoring – Week 2
During week 2, the students guided by Worksheet 2 (Supporting File S2. QTLs of Maize Cold Response – Student Handout) observe, measure, and record the level of seedling resistance to cold stress. The laboratory series implements two different approaches to documenting the level of resistance to cold stress. In the first approach that was termed qualitative, students rank the level of resistance at 1–3 point scale. In the second approach, leaf concentration of chlorophyll A and B were measured using a simple and straightforward procedure described in the student handout (Supporting File S2. QTLs of Maize Cold Response – Student Handout). Some students decided to measure the area of the leaf that was dry and used Image analysis software to produce the data. The main outcome of Week 2 is to measure and record diversity of maize seedlings response to cold stress in RILs. While student groups choose the approach to measure the trait, all students participate in data collection as the data for all lines are needed for the QTL analysis.
QTL Analysis and Interpretation of the results – Week 3
The QTL analysis approach implemented in this laboratory series relies on the use of an R package called QTL (43). In our experience, students with no or very limited knowledge of R are completely capable of running the analysis. Students were working on laboratory computers or on their own laptops, usually in groups of 2 or individually. To facilitate this step, students were asked during Week 2 to install R and a QTL package on their computers. The primary difficulty that students face is the ability to navigate the file system in R and open the data file correctly. After this step, the analysis ran smoothly. The sequence of commands was adopted from the manual for the package and is described in detail in Worksheet 3 of the student handout (Supporting File S2. QTLs of Maize Cold Response – Student Handout). Students analyze the quality of the data and explain the steps of the analysis, conduct QTL analysis using the stress response data on RILs, and build graphs presenting their data. The results are invariably different for students using different measurements for the traits. For example, the QTL analysis of qualitative data of stress response of 95 RILs and 1957 markers distributed throughout maize 10 chromosomes allowed to identify two major QTLs, on chromosomes 1 and 5 (Figure 4).
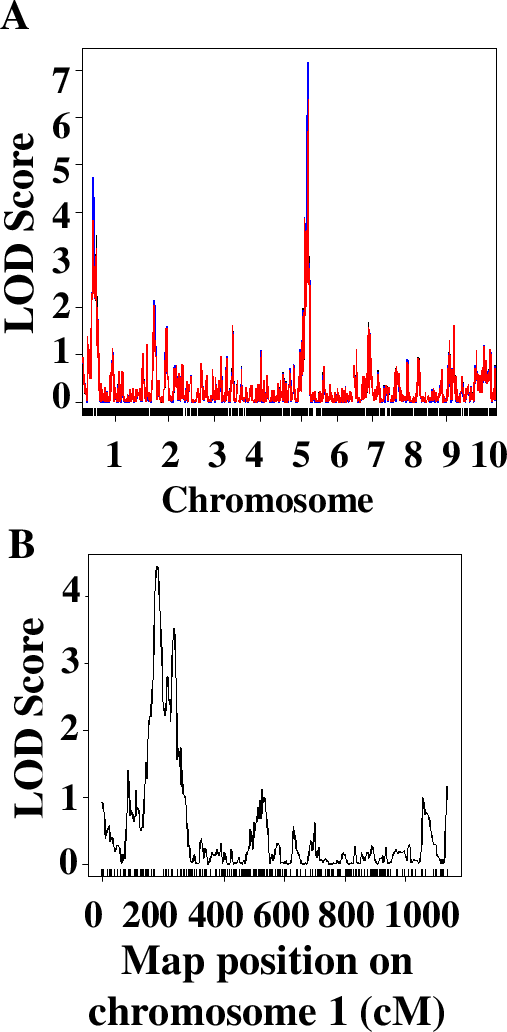
After conducting an initial analysis, students discussed and completed a series of questions analyzing the size and significance of identified QTLs and connecting physical and genetic maps of the maize genome. Specifically, they were asked to comment on the significance of the LOD score – a measure of significance of association between the locus (region of the chromosome) and the phenotypic trait (cold resistance). Students were asked to find the markers located close to their positions of the QTLs they identified using their data genetic map file (Supporting File S7. QTLs of Maize Cold Response – Sample Phenotypic Data Collected by Students) and determine their physical location using Maize Genetics Database (Supporting File S2. QTLs of Maize Cold Response – Student Handout.) Whole class discussion of students’ answers allowed for further clarifications of similarities and differences between molecular and genetic maps.
Finally, students chose one of the parameters in the QTL analysis and modified it to understand the role of this parameter in the ability of QTL analysis to identify significant QTLs. Students could choose to reduce the number of RILs or number of markers involved in the analysis, or to modify the phenotype scoring systems (1–2 instead of 1–3, for example). They modified the original data file accordingly and re-ran the analysis with their modified data. Since different groups chose to investigate different parameters, students presented their results as short chalk talks. Typically, the decreasing number of lines or markers significantly decreased the ability of the QTL analysis to identify QTLs (see Figure 5, for example). Interestingly, even employing 1–3, or even 1–2, phenotypic scoring system resulted in successful identification of QTLs, and using chlorophyll concentration measurements did not significantly enhance the quality or the number of identified QTLs. Discussing questions in the Worksheets 3 and 4 in small groups and as a whole class clarified questions and prepared them for writing lab reports. As a final assessment, students were asked to complete lab reports in the form of a scientific paper, complete with figures and figure legends. These reports were graded to evaluate student understanding of the principles of QTL analysis.
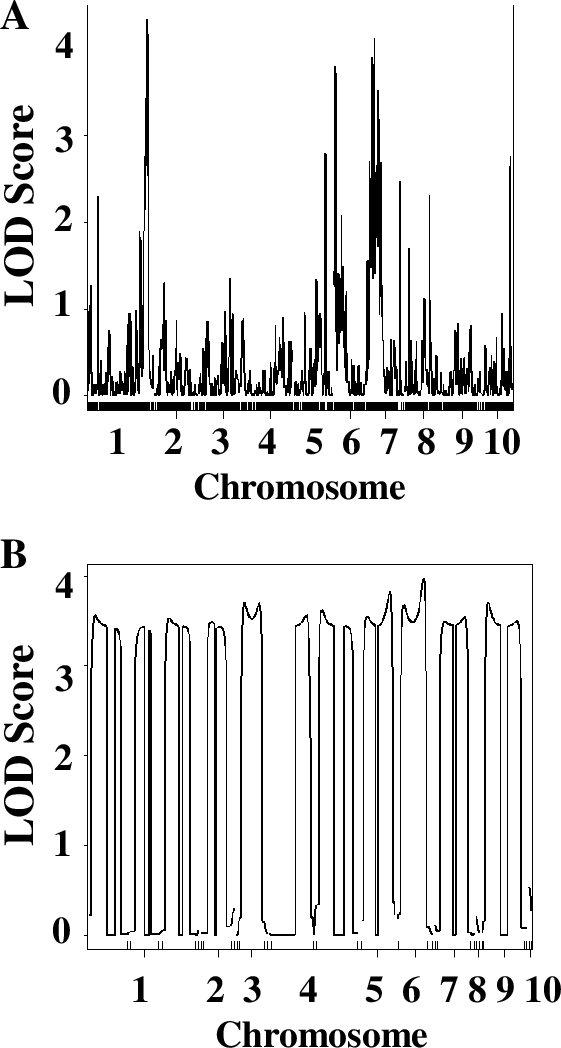
Teaching Discussion
Merits of the Laboratory Series
This laboratory series, even though relatively short, implements many aspects of the CUREs and could be extended to include additional primary literature investigations, formal presentations of students’ work, and built-in “catch up and re-do” sessions. During three three-hour lab sessions, students design experimental conditions and approaches to score the phenotypes, conduct the experiments and observations, and perform QTL analysis. While this laboratory combines a series of wet lab hands-on steps and computational data analysis, the previously collected data sets could be used in online settings or as a face-to-face computer only exercise.
Instructor Perspectives
We found that the whole group discussions, both at the early stages of the experiment planning and after students shared their results, are very important because they allow for integrating information and discovering misconceptions that could persist after small group discussions. Students frequently asked their instructor how cold stress is controlled in maize and how many genes they were supposed to find in their analysis and were empowered when the instructor communicated that nobody knows the answer. Reminding students of the importance of finding cold tolerant genes in maize and stressing the truly investigative nature of this lab series worked well to motivate students during the labs.
Evidence of Impact
Based on the results of the assessment we conducted (83 students completed the assessments in two course sections taught in different years), students demonstrated strong learning gains on the quantitative genetics quiz. Although it is difficult to separate learning in the laboratory from learning in the concurrent course, in our implementation, the average proportion of correct answers increased from 23 to 77%. For assessment of student skills in graphical data visualization and interpretation, 24 group lab reports were assessed using the rubric that focused on the appropriateness, clarity, and quality of the figures, figure legends, and data interpretations (S5. QTLs of Maize Cold Response – Rubric Used for the Assessment of Student Skills in Data Analysis and Interpretation in the Lab Reports). Only 3 of 24 lab reports (12.5%) failed to achieve the level of “accomplished” (15/20 points), while six reports (25%) scored 19 or 20 points. Average scores in all five rubric categories exceeded the level of “accomplished” (3/4 points), demonstrating that the students were able to state appropriate experimental questions, build adequate data visualizations, and interpret the results of their experiments. Finally, although this activity is not a full CURE experience, our students demonstrated gains in CURE survey categories related to understanding the scientific process (3.4 to 3.6 on a 5 point scale with a score of 0 corresponding to no perceived gain and a score of 5 as a largest possible value) and perceived skills in data analysis (3.3 to 3.7).
Possible Extensions and Modifications
Depending on the design and instructor’s preferences different groups could be conducting their own experiments or the whole class could contribute data to the shared project. In our implementations, students investigated cold stress response using quantitative scoring, image analysis, and chlorophyll concentration measurements; however, other stress conditions, such as drought and heat exposure, as well as UV exposure, provide sufficient variation between B73 and Mo17 maize inbreds and RILs for the analysis. This approach allows different groups of students to conduct novel experiments, varying the specific details of the stress conditions and plant age at the application of the stress, specific ways to measure phenotype, and the number of lines used in the analysis. In addition, other environmental factors, such as planting density, humidity, water saturation of soil, and exact timing of the stress can affect the results of the analysis. In our limited experience of testing some of these conditions, while some QTLs were detected under multiple conditions, some QTLs were not or demonstrated a different level of significance. These observations are consistent with literature on QTL analysis of cold stress response where different QTLs were reported in different studies depending on specific experimental conditions. Comparing the results from different experiments provides rich material for discussions of the limitations and benefits of quantitative genetics, scientific process and reproducibility of data, complexity of such genetic traits as stress response, and interactions between inherited genetic information and environment.
SUPPORTING MATERIALS
- S1. QTLs of Maize Cold Response – Detailed Timeline
- S2. QTLs of Maize Cold Response – Student Handout
- S3. QTLs of Maize Cold Response – Sample Answers to the Questions of the Worksheets
- S4. QTLs of Maize Cold Response – Multiple Choice Assessment Quiz with Correct Answers
- S5. QTLs of Maize Cold Response – Rubric Used for the Assessment of Student Skills in Data Analysis and Interpretation in the Lab Reports
- S6. QTLs of Maize Cold Response – Genetic Architecture of the Lines Used in This Study
- S7. QTLs of Maize Cold Response – Sample Phenotypic Data Collected by Students
- S8. QTLs of Maize Cold Response – Sample Formatted Data File Ready with Visual Scoring Data for QTL Analysis
Acknowledgments
The authors are grateful to Pete Hermanson and Nathan Springer (University of Minnesota) for providing maize seeds and to Kathryn Malody (Hamline University) for supporting our work. The authors are also grateful to many students in Principles of Genetics course for participating in this laboratory.
References
- National Research Council. BIO2010: Transforming undergraduate education for future research biologists. The National Academies Press; Washington, DC: 2003.
- American Association for the Advancement of Science. Vision and change in undergraduate biology education: A call to action. Washington, DC: 2011. [Accessed 23 February 2021]. Retrieved from: http://visionandchange.org/files/2011/03/Revised-Vision-and-Change-Final-Report.pdf.
- President’s Council of Advisors on Science and Technology. Engage to excel: Producing one million additional college graduates with degrees in science, technology, engineering, and mathematics. 2012. [Accessed 23 February 2021]. Retrieved from: www.whitehouse.gov/sites/default/files/microsites/ostp/pcast-engage-to-excel-final_feb.pdf
- Lopatto D. Survey of undergraduate research experiences (SURE): First findings. 2004 CBE Life Sci Educ. 3:270–277. doi: 10.1187/cbe.04-07-0045.
- Russell SH, Hancock MP, McCullough J. 2007. Benefits of undergraduate research experiences. Science. 316:548–549. doi: 10.1126/science.1140384.
- Laursen SL, Hunter AB, Seymour E, Thiry H, Melton G. 2010 Undergraduate research in the sciences: Engaging students in real science. John Wiley & Sons; San Francisco, CA
- Corwin LA, Graham MJ, Dolan EL. 2015. Modeling course-based undergraduate research experiences: An agenda for future research and evaluation. CBE Life Sci Educ. 14:es1. doi: 10.1187/cbe.14-10-0167.
- Rodenbusch SE, Hernandez PR, Simmons SL, Dolan EL. 2016. Early engagement in course-based research increases graduation rates and completion of science, engineering, and mathematics degrees. CBE Life Sci Educ. 15(2):ar20. doi: 10.1187/cbe.16-03-0117.
- Corwin LA, Runyon CR, Ghanem E, Sandy M, Clark G, Palmer GC, Reichler S, Rodenbusch SE, Dolan EL. 2018. Effects of discovery, iteration, and collaboration in laboratory courses on undergraduates’ research career intentions fully mediated by student ownership. CBE Life Sci Educ. 17:ar20. doi: 10.1187/cbe.17-07-0141.
- Gin LE, Rowland AA, Steinwand B, Bruno J, Corwin LA. Students who fail to achieve predefined research goals may still experience many positive outcomes as a result of CURE participation. 2018. CBE Life Sci Educ. 17(4):ar57. doi: 10.1187/cbe.18-03-0036.
- Cooper KM, Blattman JN, Hendrix T, Brownell SE. 2019. The impact of broadly relevant novel discoveries on student project ownership in a traditional lab course turned CURE. CBE Life Sci Educ. 18(4):ar57. doi:10.1187/cbe.19-06-0113
- Weaver GC, Russell CB, Wink DJ. 2008 Inquiry-based and research-based laboratory pedagogies in undergraduate science. Nat Chem Biol. 4:577–580. doi: 10.1038/nchembio1008-577.
- Schultz PW, Hernandez PR, Woodcock A, Estrada M, Chance RC, Aguilar M, Serpe RT. 2011 Patching the pipeline reducing educational disparities in the sciences through minority training programs. Educ Eval Policy Anal. 33:95–114. doi: 10.3102/0162373710392371.
- Wei CA, Woodin T. 2011 Undergraduate research experiences in biology: Alternatives to the apprenticeship model. CBE Life Sci Educ. 10:123–131. doi: 10.1187/cbe.11-03-0028.
- Auchincloss LC, Laursen SL, Branchaw JL, Eagan K, Graham M, Hanauer DI, Lawrie G, McLinn CM, Pelaez N, Rowland S, Towns M, Trautmann NM, Varma-Nelson P, Weston TJ, Dolan EL. 2014 Assessment of course-based undergraduate research experiences: A meeting report. CBE Life Sci Educ. 13:29–40. doi: 10.1187/cbe.14-01-0004.
- Bangera G, Brownell SE. 2014. Course-based undergraduate research experiences can make scientific research more inclusive. CBE Life Sci Educ. 13:602–606. doi: 10.1187/cbe.14-06-0099.
- Corwin LA, Runyon C, Robinson A, Dolan EL. 2015. The laboratory course assessment survey: A tool to measure three dimensions of research-course design. CBE Life Sci Educ. 14:ar37. doi: 10.1187/cbe.15-03-0073.
- van Eeuwijk FA, Bink MC, Chenu K, Chapman SC. 2010. Detection and use of QTL for complex traits in multiple environments. Curr Opin Plant Biol. 13(2):193-205. doi: 0.1016/j.pbi.2010.01.001.
- Barroso I, McCarthy MI. 2019. The genetic basis of metabolic disease. Cell. 177(1):146-161. doi: 10.1016/j.cell.2019.02.024.
- Frazer KA. 2009. Human genetic variation and its contribution to complex traits. Nat Rev Gen. 10:241. doi: 10.1038/nrg2554.
- Marbach-Ad G. 2004. Expectations and difficulties of first year college students in biology. Journal of College Science Teaching, 33, 18-23.
- Uno GE. 2009. Botanical literacy: What and how should students learn about plants? American Journal of Botany 96:1753-1759. doi: 10.3732/ajb.0900025.
- Wandersee J, Schussler E. 1999. Preventing plant blindness. The American Biology Teacher 61:82–86.
- Parsley, KM. 2020. Plant awareness disparity: A case for renaming plant blindness. Plants, People, Planet. 2020;2:598–601.
- Balding M, Williams KJ. 2016. Plant blindness and the implications for plant conservation. Conserv Biol. 30(6):1192-1199. doi: 10.1111/cobi.12738.
- Gelvin SB, Karcher SJ. 1996. Reporter genes and transgenic plants to study response to environmental signals. pp:71-84, in Tested studies for laboratory teaching, Volume 17 .
- Wendell DL, Pickard D. 2007. Teaching human genetics with mustard: rRapid cycling Brassica rapa (fast plants type) as a model for human genetics in the classroom laboratory. CBE Life Sci Educ 6:179–185. doi:10.1187/cbe.07-02-0010
- Hacisalihoglu G, Hilgert U, Nash EB, Micklos DA. 2008. An innovative plant genomics and gene annotation program for high school, community college, and university faculty. CBE Life Sci Educ 7:310 –316.
- Chrispeels HE, Klosterman ML, Martin JB, Lundy SR, Watkins JM, Gibson CL, Muday JK. 2014. Undergraduates achieve learning gains in plant genetics through peer teaching of secondary students. CBE Life Sci Educ 13:641–652. doi: 10.1187/cbe.14-01-0007.
- Murren C J, Wolyniak MJ, Rutter MT, Bisner AM, Callahan HS, Strand AE, Corwin LA. 2019. Undergraduates phenotyping Arabidopsis knockouts in a course-based undergraduate research experience: Exploring plant fitness and vigor using quantitative phenotyping methods. J Microbiol Biol Educ. 20(2):20.2.28. doi: 10.1128/jmbe.v20i2.1650.
- Zhu JK. 2016. Abiotic stress signaling and responses in plants. Cell, 167(2), 313–324. Doi: 10.1016/j.cell.2016.08.029
- Baillo EH, Kimotho RN, Zhang Z, Xu P. 2019. Transcription factors associated with abiotic and biotic stress tolerance and their potential for crops improvement. Genes (Basel). 10(10):771. doi: 10.3390/genes10100771.
- Erpen L, Devi HS, Grosser JW, Dutt M. 2018. Potential use of the DREB/ERF, MYB, NAC and WRKY transcription factors to improve abiotic and biotic stress in transgenic plants. 2018. Plant Cell Tissue Organ Cult. 132:1–25. doi: 10.1007/s11240-017-1320-6.
- Ma H, Liu C, Li Z, Ran Q, Xie G, Wang B, Fang S, Chu J, Zhang J. 2018. ZmbZIP4 contributes to stress resistance in maize by regulating ABA synthesis and root development. Plant Physiol. 178(2):753-770. doi: 10.1104/pp.18.00436.
- Leng P, Zhao J. 2020. Transcription factors as molecular switches to regulate drought adaptation in maize. Theor Appl Genet. 133(5):1455-1465. doi: 10.1007/s00122-019-03494-y.
- Jompuk C, Fracheboud Y, Stamp P, Leipner J. 2005. Mapping of quantitative trait loci associated with chilling tolerance in maize (Zea mays L.) seedlings grown under field conditions. J Exp Bot. 56(414):1153-63. Doi: 10.1093/jxb/eri108.
- Rodríguez VM, Butrón A, Rady MOA, Soengas P, Revilla P. 2014. Identification of quantitative trait loci involved in the response to cold stress in maize (Zea mays L.). Mol Breeding. 33:363–371.
- Hu S, Lübberstedt T, Zhao G, Lee M. 2016. QTL mapping of low-temperature germination ability in the maize IBM Syn4 RIL population. PLoS One. 11(3):e0152795. doi:10.1371/journal.pone.0152795.
- Revilla P, Rodríguez VM, Ordás A, Rincent R, Charcosset A, Giauffret C, et al. 2016. Association mapping for cold tolerance in two large maize inbred panels. BMC Plant Biol. 16(1):127. doi: 10.1186/s12870-016-0816-2.
- Yi Q, Malvar RA, Álvarez-Iglesias L, Ordás B, Revilla P. 2020. Dissecting the genetics of cold tolerance in a multiparental maize population. Theor Appl Genet. 133(2):503-516. doi: 10.1007/s00122-019-03482-2.
- Lee M, Sharopova N, Beavis WD, Grant D, Katt M, Blair D et al. 2002. Expanding the genetic map of maize with the intermated B73 × Mo17 (IBM) population. Plant Mol Biol. 48:453–461.
- Miles C and Wayne M. 2008. Quantitative trait locus (QTL) analysis. Nature Education 1(1):208.
- Broman KW, Wu H, Sen Ś, Churchill GA. 2003. R/qtl: QTL mapping in experimental crosses. Bioinformatics. 19:889-890.
- Lopatto D, Alvarez C, Barnard D, Chandrasekaran C, Chung HM, Du C, et al. 2008. Undergraduate research. Genomics education partnership. Science. 322(5902):684-685. doi: 10.1126/science.1165351.
Article Files
Login to access supporting documents
Makarevitch-Learning quantitative genetics.pdf(PDF | 356 KB)
S1. QTLs of Maize Cold Response - Timeline.docx(DOCX | 18 KB)
S2. QTLs of Maize Cold Response - Student Handout.docx(DOCX | 33 KB)
S3. QTLs of Maize Cold Response - Sample Student Answers.docx(DOCX | 272 KB)
S4. QTLs of Maize Cold Response - QTL Mapping Quiz.docx(DOCX | 42 KB)
S5. QTLs of Maize Cold Response - QTL Rubric Lab Report.docx(DOCX | 21 KB)
S6. QTLs of Maize Cold Response - QTL Genetics of the RILs.csv(CSV | 401 KB)
S7. QTLs of Maize Cold Response - QTL Formatted Data File.csv(CSV | 416 KB)
S8. QTLs of Maize Cold Response - QTL Phenotypic Data.csv(CSV | 2 KB)
- License terms
Comments
Comments
There are no comments on this resource.